QuanCrypt-FL: Quantized Homomorphic Encryption with Pruning for Secure Federated Learning
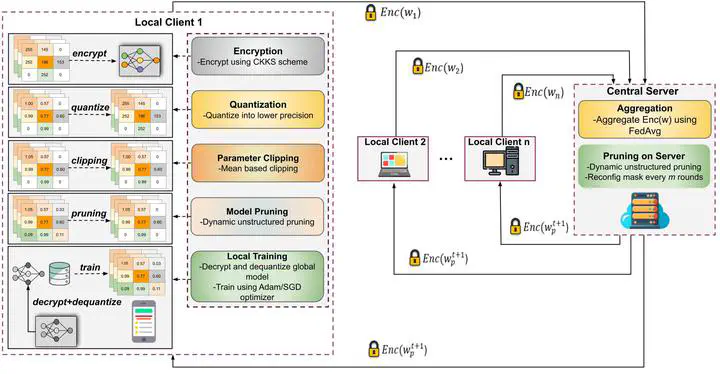
QuanCrypt-FL is a communication-efficient federated learning algorithm that integrates low-bit quantization, pruning, and mean-based clipping to enhance privacy protection against inference attacks while minimizing computational costs and maintaining high model accuracy. Validated on MNIST, CIFAR-10, and CIFAR-100 datasets, QuanCrypt-FL outperforms state-of-the-art methods, achieving significantly faster encryption, decryption, and training times compared to BatchCrypt.