CorBin-FL
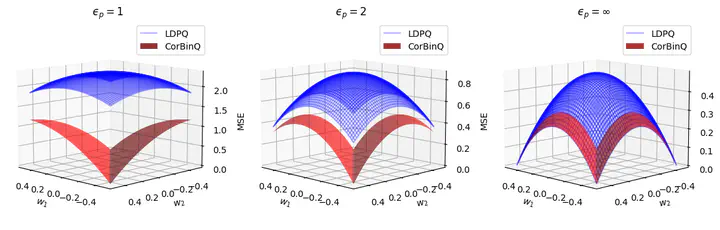
Federated learning (FL) enables collaborative model training among distributed clients, but it faces challenges in balancing privacy, communication efficiency, and model accuracy. CorBin-FL is introduced as a privacy mechanism that employs correlated binary stochastic quantization to achieve differential privacy while preserving high model performance. The method uses secure multi-party computation techniques to enable clients to quantize their local model updates without revealing private data. Theoretical analysis shows that CorBin-FL provides parameter-level local differential privacy (PLDP) and optimizes the trade-off between privacy and utility, measured by mean squared error and PLDP guarantees. An extension, AugCorBin-FL, is proposed to further enhance privacy at the user and sample levels, offering central differential privacy in addition to PLDP. Experiments on MNIST and CIFAR-10 datasets show that both mechanisms outperform existing privacy-preserving FL approaches, such as Gaussian and Laplacian mechanisms, in terms of model accuracy under the same privacy budgets.